Demystifying Machine Learning for Non-Programmers

In the rapidly evolving landscape of the digital world, one term that has gained significant traction is machine learning. With its potential to transform industries and revolutionize processes, it has become a key focus for organizations across the globe. But what exactly is machine learning, and how does it work? In this article, we will delve into the depths of machine learning, shedding light on its intricacies in a manner accessible to beginners and non-technical readers.
Unveiling the Inner Workings
At its core, machine learning is a branch of artificial intelligence (AI) that empowers systems to learn and make decisions without explicit programming. Unlike traditional rule-based approaches, machine learning algorithms rely on patterns and data to improve their performance over time. Through a series of interconnected steps, machine learning unlocks valuable insights and predictions, driving automation and efficiency.
The journey of machine learning can be divided into several stages, each playing a pivotal role in the process. It begins with data collection, where a rich and diverse dataset is assembled, serving as the foundation for training the machine learning model. This data serves as the building blocks for the algorithms to recognize patterns and relationships, enabling them to make informed decisions.
Following data collection, the next step is data preprocessing. In this crucial stage, the collected data undergoes cleaning and transformation. Inconsistencies, outliers, and missing values are addressed, ensuring that the data is in a suitable format for the algorithms to digest. This preparatory phase sets the stage for robust and accurate model training.

The heart of machine learning lies in model training. Here, the preprocessed data is fed into the machine learning model, allowing it to learn from the underlying patterns within the dataset. Through an iterative process, the model adjusts its internal parameters, minimizing errors and improving its predictive capabilities. The more comprehensive and representative the data, the more powerful and accurate the model becomes.
Once the model has been trained, it undergoes evaluation. A separate dataset, known as the test set, is employed to assess the model's performance. This evaluation ensures that the model's predictive abilities extend beyond the training data, allowing it to generalize and provide accurate insights on unseen data.
Why is Machine Learning Helpful or Useful?
Machine learning offers numerous benefits and applications that make it a powerful tool across various domains:
Automation and Efficiency: Machine learning streamlines complex tasks and decision-making processes, leading to enhanced efficiency and productivity. By swiftly analyzing massive volumes of data, organizations can make data-driven decisions faster and with greater accuracy, ultimately saving time and resources.
Pattern Discovery: Machine learning algorithms possess an innate ability to uncover hidden patterns and relationships within data. This capacity allows organizations to extract valuable insights, identify trends, and predict future outcomes. By harnessing this power, businesses can make informed decisions and gain a competitive edge.
Personalization: Machine learning enables personalized experiences by deeply understanding individual preferences and behaviors. By analyzing vast amounts of data, organizations can deliver tailored recommendations, customized user experiences, and targeted advertising. This personalization fosters stronger customer engagement, loyalty, and satisfaction.
Fraud Detection and Cybersecurity: With the increasing prevalence of fraudulent activities and cybersecurity threats, machine learning provides a robust line of defense. By continuously monitoring data streams and identifying anomalous patterns, machine learning algorithms can swiftly detect potential fraud and security breaches. This proactive approach mitigates risks and safeguards critical systems and information.
What is the Future of Machine Learning?
As we peer into the future, it becomes evident that machine learning is poised for remarkable advancements. Here are a few key areas that will shape the future of machine learning:
Advancements in Deep Learning: Deep learning, a subfield of machine learning, has been instrumental in recent breakthroughs. As we move forward, deep learning architectures will continue to evolve, enabling more accurate and sophisticated models. This will empower machines to comprehend and process complex data, expanding the application of machine learning across diverse industries.
Reinforcement Learning: Reinforcement learning, a branch of machine learning focused on decision-making in dynamic environments, will witness significant advancements. With continuous learning through trial and error and receiving rewards or punishments based on actions, reinforcement learning will enable machines to adapt and make optimal decisions in real-time. This capability will find applications in areas that demand constant interaction and decision-making.
Unsupervised Learning: Unsupervised learning, where algorithms learn from unlabeled data to discover patterns and structures, will continue to advance. This approach enables machines to uncover hidden relationships and generate insights without explicit guidance. The future will bring forth more sophisticated unsupervised learning techniques, allowing machines to extract valuable information from vast amounts of unstructured data, enhancing decision-making and problem-solving abilities.
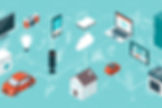
Edge Computing and IoT Integration: With the proliferation of Internet of Things (IoT) devices, there will be an increasing need for machine learning models to be deployed on the edge, closer to the data source. Edge computing enables real-time data processing and analysis, reducing reliance on cloud-based systems and minimizing latency. In the future, machine learning models will be designed to efficiently run on resource-constrained edge devices, facilitating faster and more intelligent decision-making at the network's edge.
Ethical Considerations and Fairness: As machine learning becomes more ubiquitous, ethical considerations and fairness will be at the forefront of development. The awareness of biases embedded in machine learning algorithms and the potential for discriminatory outcomes has grown. In the future, there will be a concerted effort to address these biases and ensure fairness, transparency, and accountability in the development and deployment of machine learning models.
Embracing the Power of Machine Learning
To harness the benefits of machine learning in a pragmatic way, both businesses and individuals can take proactive steps. Here are some approaches to adopt machine learning in a meaningful manner:
Embrace a Data-Driven Culture: Start by cultivating a data-driven mindset within your organization. Recognize the value of data and invest in its collection, organization, and analysis. By leveraging machine learning techniques, you can derive meaningful insights and make informed decisions based on data rather than intuition or guesswork.
Identify Pain Points and Opportunities: Assess your business processes and identify areas where machine learning can add value. Look for tasks that are repetitive, time-consuming, or require complex data analysis. By automating such processes with machine learning, you can streamline operations, improve efficiency, and focus resources on higher-value activities.
Collaborate with Experts: Engage with experts in the field of machine learning to gain insights and guidance. Partner with data scientists or hire machine learning specialists to help you navigate the complexities of implementing machine learning in your organization. Their expertise can ensure a smooth transition and maximize the benefits of this technology.
Invest in Infrastructure and Resources: Building a robust infrastructure and allocating resources for machine learning initiatives is crucial. Ensure that you have the necessary computational power, storage, and software tools to support the training and deployment of machine learning models. Additionally, invest in training and upskilling your team to develop a data-literate workforce capable of harnessing the power of machine learning.
Stay Informed and Adapt: The field of machine learning is ever-evolving, with new techniques, algorithms, and applications emerging regularly. Stay up to date with the latest advancements, industry trends, and best practices. Attend conferences, participate in webinars, and engage with the machine learning community to stay ahead of the curve.
Wrap-Up
Machine learning has become a transformative force in today's digital landscape. By enabling systems to learn from data and make informed decisions, it offers numerous benefits across various industries. As machine learning continues to evolve, advancements in deep learning, reinforcement learning, unsupervised learning, edge computing, and ethical considerations will shape its future. To take advantage of this technology, businesses and individuals can embrace a data-driven culture, identify pain points and opportunities, collaborate with experts, invest in infrastructure and resources, and stay informed and adaptable.
Machine learning is a powerful tool that holds immense potential for organizations and individuals alike. By understanding its inner workings and staying abreast of technological advancements, you can leverage machine learning to drive innovation, enhance decision-making, and gain a competitive edge. Whether you're a business owner seeking process automation or an individual interested in the impact of AI on daily life, machine learning has the potential to revolutionize the way we work, live, and interact with technology. So embrace the power of machine learning and embark on a journey of discovery and transformation!
To explore partnering with experts to leverage machine learning for your business, book a free consultation with Render Analytics. To learn about some common data science use cases for businesses, give this article a read!